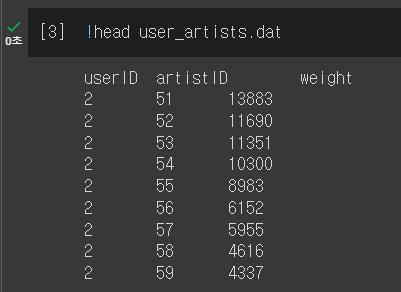
L05.1 User-free Models Practice
·
AI/추천 시스템 설계
last.fm 데이터셋● last.fm: 온라인 음악 Database, 추천 서비스● HetRec 2011 에서 데이터셋 공개 ○ 92,800 artists, 1892 users ○ https://grouplens.org/datasets/hetrec-2011/!wget https://files.grouplens.org/datasets/hetrec2011/hetrec2011-lastfm-2k.zip!unzip hetrec2011-lastfm-2k.zip 데이터 준비● artists 정보 불러오기artists = {}with open('artists.dat', 'r') as f: print(f.readline()) for line in f: id, name, _, _ = l..